All AI and CX projects need structured data. Regardless of the phase and organizational hierarchy, there are 4 principles health organisations must adhere to for effective data governance.
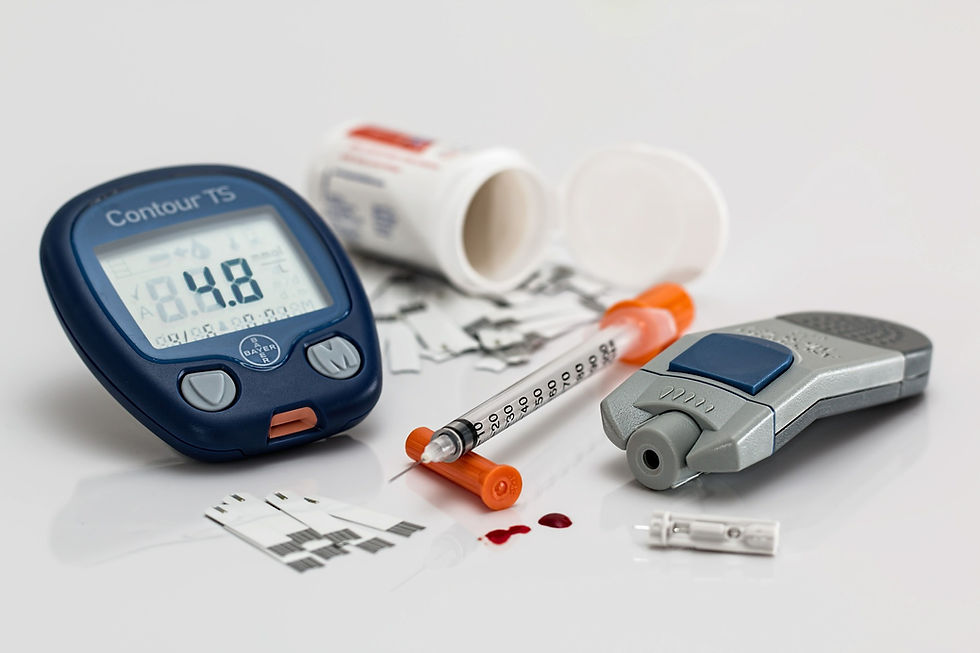
Evaluating the 4 principles for effective data governance:
Data Value: Organisations need to make stakeholders understand data is a strategic asset, it’s value must be unequivocally embedded across the whole organization from the board – to all functional team members.
Data Culture: Building a data-driven culture is a meticulous task, one that is well rewarded over time. Health organisations must attach goals and milestones around data acquisition when embedding a data-first approach within their departments. For good data governance, the data must be compliant as per GDPR and other regulatory guidelines.
Organisational Alignment: The data governance policy must articulate and support the strategic priorities of the health organisation. Data must be captured and stored to service the needs of stakeholders, identify conflicts and opportunities, and patterns of behaviour.
Lean Methodology: Lean methodology is a good strategy to adopt. The leadership team must exercise judiciousness and evaluate the needs and set goalposts as the project advances, or the organization matures.
Data governance must be adhered to till the very end of a project if ROI is to be maximized.
Let’s discuss the action plans, including four critical steps that CX leaders may wish to consider as they plan a data-driven implementation.
Any organization that initiates a data-driven strategy will face barriers and organizational resistance. However, with a clear vision, even companies with undeveloped CX systems, restricted data, and a lack of data specialists can convert their CX programs to deliver strong customer experiences.
The efficient approach to strengthening CX is creating personalized customer journeys because the one-size-fits-all strategy is extremely outdated and not geared to deliver results.
1. Enabling access to diverse data
Data-driven companies rely on insights and information to steer growth. They utilize their big data to create value and aim for operational excellence to streamline processes and that enhance seamless service delivery. Volumes of structured data are analysed to draw inferences including data from business transaction systems, customer databases (such as purchase behaviour and preferences), clickstream logs (digital behaviours), social media activity, medical records, and others. The patterns from the data analysed allow the company to improve its operations, enhance customer service, personalise marketing campaigns, and increase profitability.
2. Predictive modelling
Further, refining and leveraging data to aid decision-making allows alignment with business strategy and provides operational flexibility across departments to drive change effectively.
Whilst the specific implementation of data structures varies by organisation. the predictive modelling approach follows three core principles:
i) Customer level data sets – the customer-level data sets contain a vast amount of raw data. This is data collected from different sources such as web systems, mobile applications, social media, IoT devices, and pre-existed data run in real-time modes. The company processes raw data and stores it in a cloud-based platform where encryption supports and compresses it in a cost-effective manner. After data is processed, the comprehensive customer-level data sets allow for an in-depth analysis of interactions, transactions, and operations to help refine customer journeys.
ii) Predictive customer scores – By leveraging data analytics and machine learning tools, the customer level data sets can deliver actionable insights into customer satisfaction, business performance, or predicting an accurate outcome. Algorithms can give predictions with a high probability of occurrence when substantial data is available from past events. This allows CX leaders to determine ROI for particular investments and work with variables that are most likely to produce desired business outcomes.
iii) Database Management System (DBMS) – this step requires the software to serve as an interface between databases and employees, ensuring that data is constantly organized to create, protect, read, and update to allow personalized customer experiences and improve CX outcomes. The data collection allows predictive feedback that companies can use to measure their CX performance; they also improve strategic decision-making.
3. Predictive analytics enabling the omnichannel strategy
Organisations have embraced the omnichannel strategy pretty well to boost performance, even though digital channels were highly accelerated during the pandemic. The ‘back to basics' approach has been adopted by a lot of organisations when formulating their omnichannel strategy - constructing customer data sets to identify, correct, record and influence customer satisfaction and business performance across priority customer journeys. By leveraging predictive analytics organisations can create interactions with the customer, both current and potential, at all customer-facing touchpoints: organizing, automating and synchronizing when interpreting outcomes and responses. Whether it be a website, social media, online ads, a retail interaction, or an online purchase, predictive analytics can level up the ability to direct customer insights for recommending actionable improvements in the digital customer journey.
4. Predictive CX Platform – Statistics-driven analysis allows organisations to see the qualified and organized view into the difficulties, prospect areas, and channel interactions across millions of customers, enabling them to support a recurring journey-improvement cycle. By combining data collection processes, analytics, and machine learning algorithms, business leaders can use data from the past to predict the future. Thus, CX transformation should focus on tracking customer behaviour online, raising red flags in a customer journey, assessing missed opportunities, this will allow predictive analytics to suggest personalized digital experience solutions to supercharge customer retention.
The system can also be recognized as a survey engine to collect valuable customer feedback across all customer touchpoints and collate the responses into the CX platform. Through these mechanisms, the team can use the analytics platform to achieve cost savings and revenue growth eg reducing interaction and operation costs by 10 to 25 per cent due to CX and digital transformation.
Consumer trends are shifting continually and businesses must adapt to the changing needs post-pandemic. This allows you to improve not only the customer experience but also build customer loyalty. Creating a better customer experience positions your organization to be innovative, intelligent, and better suited to survive in a fast-changing environment.
Change management must ensure human experience becomes a culture organization-wide. Read on.
Comentários